AI Powers Advances in Preclinical Imaging Analysis
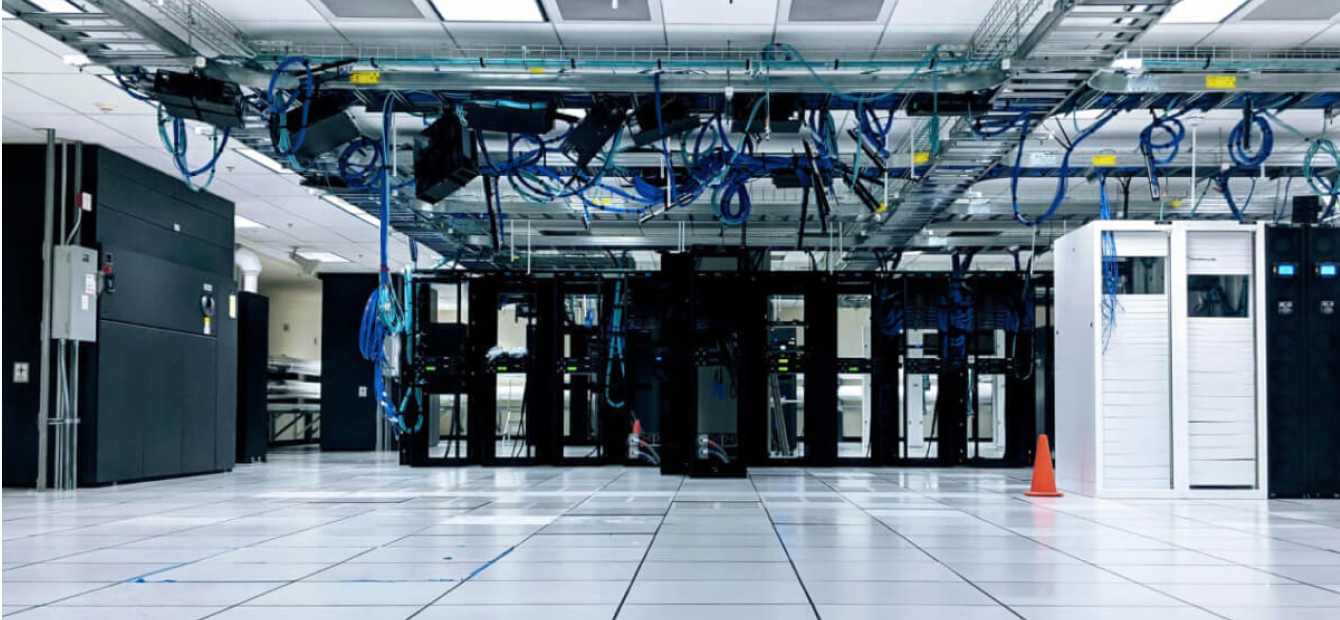
Preclinical imaging is already a powerful research and diagnostic tool. Now studies using CT, MRI, PET, and optical imaging technologies among others, are playing an important role in drug discovery and development. Preclinical imaging can save the pharmaceutical industry time and money by assessing the effects of drug candidates earlier in the drug pipeline – ultimately helping to get new drugs to patients faster. Applying artificial intelligence to image data analysis is advancing the field, allowing the development of innovative solutions where biology, physics, and computer science intersect.
Machine learning meets biology
The use of machine learning in image data analysis and visualization is rapidly advancing the value of preclinical imaging in drug development. Machine learning is a branch of artificial intelligence (AI) in which algorithms can “learn” from data by identifying patterns, using structured data sets to modify themselves in order to achieve a certain output. (For a popular culture example, see the 1983 film “WarGames,” in which a computer controlling the world’s nuclear arsenal repeatedly played simple tic-tac-toe games against itself until it “learned” that nuclear war is always a “no-win” situation.) A newer subset of machine learning, deep learning, uses a network of multiple algorithms that may interpret the data in different ways—this is also known as an artificial neural network.
Last year, Charles River Labs began a machine learning project “focused on lesion segmentation in the anatomical MRI data from transient medial cerebral artery occlusion model of ischemic stroke in rat,” says Artem Shatillo, head of MRI at Charles River. Starting with imaging data that contained manually delineated stroke lesions, they used a deep learning tool to teach a fully convolutional network algorithm to detect borders of ischemic areas, as well as other features.
Before the advent of machine learning, they maximized automation of the routine imaging data processing pipelines for tasks like converting, exporting, grouping, and quantifying data, so that imaging data can be batch-processed on a dedicated server with no user input. But all other data analysis tasks still required considerable amounts of manual work. With new machine learning tools, it is possible to minimize human errors and bias, and speed up the process by several orders of magnitude, according to Shatillo. “Software tools like this are dramatically accelerating data analysis, while also increasing consistency and quality of the outcome compared to manual segmentation,” he says. “In turn, this can make a difference between ‘go’ and ‘no go’ decisions for the drug candidate.”
“I believe machine learning will become an industry standard in digital imaging analysis very quickly, and we need to be prepared for that,” says Shatillo. “At Charles River, we are planning to validate and implement similar analysis tools for multiple imaging modalities.” Next they plan to use machine learning for MRI volumetry data analysis, which measures absolute volumes of brain structures, for example, to detect cortical atrophy in neurodegenerative disease models…